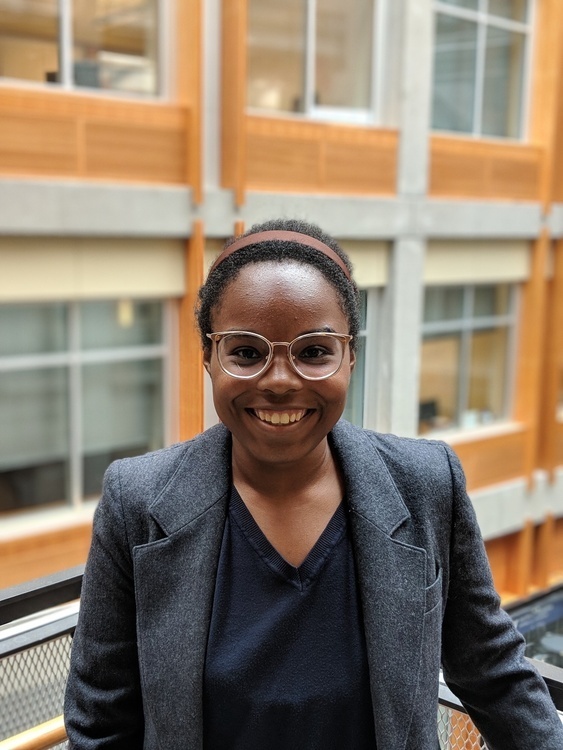
- Bill and Melinda Gates Hall, 114
Language can capture complex and pervasive social biases, which reinforce power dynamics that harm marginalized groups. In this talk, Saadia Gabriel will discuss three case studies in her research on recognizing the patterns of harmful or false language like COVID-19 (dis/mis)information and hate speech. She will also discuss progress and challenges in mitigating harmful effects on marginalized groups, and how these case studies relate to the narrative of her own life.
Saadia Gabriel is a 5th year PhD student in the Paul G. Allen School of Computer Science & Engineering at the University of Washington, where she is advised by Prof. Yejin Choi. Her research revolves around natural language understanding and generation, with a particular focus on machine learning techniques and deep-learning models for understanding how social commonsense manifests in text (i.e., how do people typically behave in common social scenarios), as well as mitigating online toxicity (e.g., hate speech) and spread of false or harmful text. Her work has been published in top NLP/AI conferences and has been nominated for several research awards and fellowships (including a 2019 ACL short paper nomination, a best paper award at the 2020 WeCNLP summit and a 2021 Google-Leap fellowship).
Lunch provided! Space is limited, registration is required.